Abstract
On the shoulders of textual dialog systems, the multimodal ones, recently have engaged increasing attention, especially in the retail domain. Despite the commercial value of multimodal dialog systems, they still suffer from the following challenges: 1) automatically generate the right responses in appropriate medium forms; 2) jointly consider the visual cues and the side information while selecting product images; and 3) guide the response generation with multi-faceted and heterogeneous knowledge. To address the aforementioned issues, we present a Multimodal diAloG system with adaptIve deCoders, MAGIC for short.
In particular, MAGIC first judges the response type and the corresponding medium form via understanding the intention of the given multimodal context. Hereafter, it employs adaptive decoders to generate the desired responses: a simple recurrent neural network (RNN) is applied to generating general responses, then a knowledge-aware RNN decoder is designed to encode the multiform domain knowledge to enrich the response, and the multimodal response decoder incorporates an image recommendation model which jointly considers the textual attributes and the visual images via a neural model optimized by the max-margin loss. We comparatively justify MAGIC over a benchmark dataset. Experiment results demonstrate that MAGIC outperforms the existing methods and achieves the state-of-the-art performance.
Model
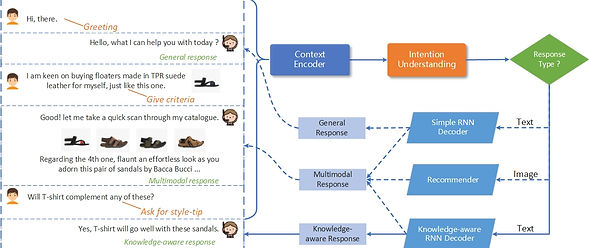
Framework

Context Encoder
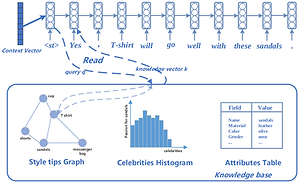
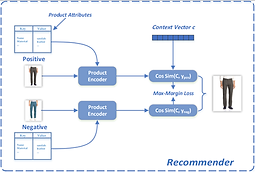
Multiform knowledge-aware RNN Decoder
Recommender
Dataset
To build task-oriented multimodal dialog systems, we utilized the MMD dataset in the retail domain to train and evaluate our proposed model MAGIC. The MMD dataset consists of over 150k conversations between users and chatbots, and each conversation comprises approximately 40 utterances.
Among them, every user’s utterance in the conversation is labeled with one of the 15 intention categories. And over 1 million fashion products with a variety of domain knowledge were crawled from several well-known online retailing websites, such as Amazon6 and Jabong7 . Different from MHRED and KMD that utilize the fixed visual features of products obtained from the FC6 layer of the VGGNet-16, we crawled the original pictures of these products from the websites to facilitate the extraction of visual features. Similar to MHRED and KMD, we treated every utterance of chatbots in the conversations as a target response and its former utterances as the context. Apart from that, we classified them into three types of responses to train the corresponding decoders separately.